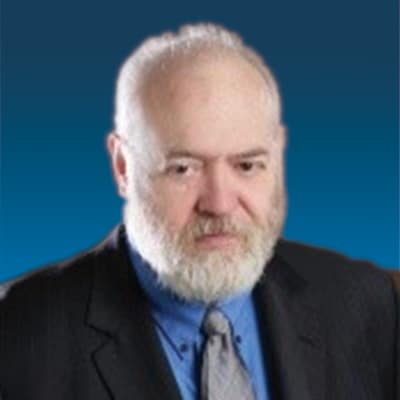
David Thomas
While AI promises utility grid operations a shiny upgrade, it’s not all sunshine and roses. The landscape is advancing exponentially as AI companies race to create a product that beats the competition. However, the technology has a host of volatilities and vulnerabilities that must be addressed.
This is part 2 of a two-part series. Read part 1 which covers AI’s potential positive grid operations impacts.
Artificial intelligence has enormous implications for the energy sector. Grids will operate far more efficiently with real-time monitoring and decision-making and enhanced predictive capabilities. However, there are two sides to this coin. While AI offers solutions and enhancements to grid operations, it comes with new problems and vulnerabilities. From faulty or biased code to a lack of understanding of how the digital mind works, myriad scenarios can make AI’s impact on the grid problematic and perhaps even catastrophic.
The problem with AI
AI promises a new era of technological innovation, but many are urging caution—some even calling for a complete halt in development. However, given the competitive landscape, slowing down is an unlikely outcome.
Why the pushback on such disruptive tech?
The issue predominantly lies in the chaotic nature inherent to artificial intelligence. AI is often described as a ‘black box’ because experts don’t always know how or why certain outputs are reached. And until we gain an understanding of these calculations and implement proper safeguards, using AI in critical infrastructure can lead to serious problems.
Regardless of popular sentiment, there are a few considerations to be had before embracing AI into grid operations.
Every silver lining has a cloud
Migrating operations to the cloud is a trend that has been around for a while. However, going digital invites a host of new problems to solve and vulnerabilities that require protection.
Data quality and integrity
Gaps in the AI training data frequently lead to “hallucination”: an AI inventing data that doesn’t exist or giving a totally invalid answer. AI responses are only as accurate or complete as the raw data in the training set. This is why selecting the training set is critically important. If the data is flawed, incomplete, or biased, it leads to incorrect conclusions and/or actions. Unchecked, these could ultimately compromise grid operations.
Unforeseen Circumstances
While AI is proficient at handling situations it’s been trained on; it may not perform well in situations that deviate from what it has encountered during its training phase. For instance, if an AI has only been trained on normal operating conditions, dealing with parameters beyond its training (i.e., extreme weather events), it may yield poor results. While we can train them for outlier situations, imbuing AI with fail-safe measures, mechanisms for human intervention, and robust recovery procedures, anticipating every possible divergent scenario is impossible.
Lack of interpretability and transparency
AI doesn’t interpret information as we do. The black box nature of AI algorithms—especially deep learning models—can be highly intricate and be composed of multiple layers of (often using thousands, if not millions, or parameters) interconnected nodes. These systems can generate predictions or reach decisions based on billions of calculations without explanation—making it impossible for experts to understand its rationale. Understanding why and how certain decisions were reached and how to fix issues is an active and exceedingly challenging area of AI research.
Cybersecurity vulnerabilities
AI systems are obvious targets for hackers seeking to disrupt grid operations. Data poisoning can severely distort AI results. Long-term exposure to corrupted data could potentially damage the underlying AI model. This is especially true for any machine learning implementation that performs ongoing training.
Humans and AI
Legislation
As with all bleeding-edge technology, legal concerns about data ownership, copyright, and liability lag far behind. EU legislation targeting AI is under consideration, but that is a topic for a later conversation.
Dependency
Running the grid is a delicate task that requires real-time monitoring and decision-making. While AI delivers that and then some, the more immersed it becomes in grid operations, the more grid operators will lean on it. If we’re not careful, we may develop an over-reliance on these systems, which can lead to complacency and a lack of healthy skepticism.
AI: a work in progress
Advancements in artificial intelligence have sparked excitement around the world. The disruptive technology can be applied to almost any practice or business. From creating detailed and realistic art to intelligent automation of complex processes, the possibilities seem limitless. Indeed, it’s only a matter of time before we implement it into our critical infrastructure. However, while AI can enhance grid operations, the technology is not without risk. Many issues still require safeguards and solutions before considering AI a viable tool for grid operations.
Want to learn more about what’s going on with grid operations? PSC covers a range of challenges and advancements in the space. Contact us!